Balancing Data Protection and Innovation under GDPR
Discover how forward-thinking organizations are successfully navigating GDPR requirements while accelerating innovation in 2025. Learn practical strategies to transform compliance challenges into competitive advantages.
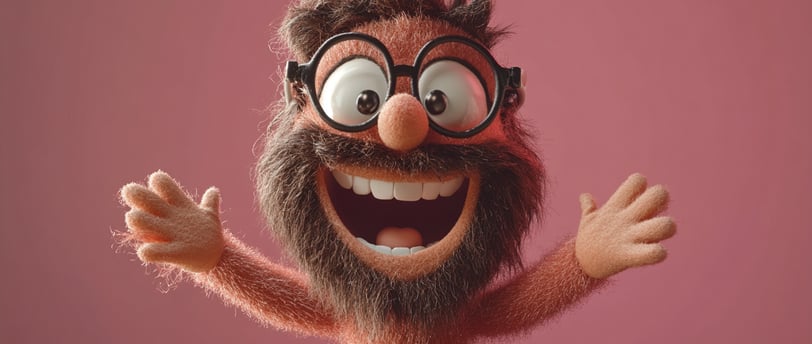
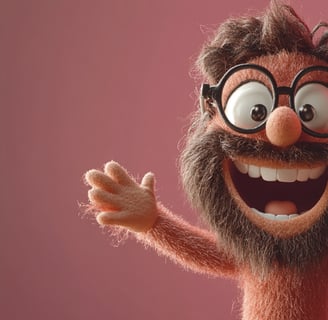
The General Data Protection Regulation (GDPR), enacted by the European Union, represents a landmark achievement in global data privacy. Its core objective is to afford individuals greater control over their personal data while standardizing data protection across the EU. Simultaneously, data-driven innovation, particularly through Artificial Intelligence (AI) and machine learning, has become an indispensable engine for economic growth, scientific advancement, and novel business opportunities. This report examines the intricate relationship between GDPR and innovation, highlighting the inherent tensions arising from the regulation's stringent principles, while also identifying significant opportunities and strategic frameworks that enable organizations to foster innovation responsibly within the GDPR landscape. While compliance costs and regulatory ambiguities present notable challenges, particularly for smaller entities, the emphasis on trust, data quality, and ethical practices embedded within GDPR can ultimately serve as a powerful catalyst for sustainable and competitive innovation.
The Dual Mandate of GDPR
The digital age is characterized by an unprecedented reliance on data, which has become a fundamental resource driving progress across various sectors. This reliance, however, necessitates robust frameworks to protect individual privacy, leading to the emergence of comprehensive regulations like the General Data Protection Regulation.
1.1 Defining GDPR and its Core Objectives
The General Data Protection Regulation (GDPR), implemented by the European Union in May 2018, stands as a pivotal legal framework governing data privacy. It establishes stringent rules for the collection, processing, and storage of personal data, aiming to empower individuals with greater control over their information. This regulation replaced the earlier 1995 Data Protection Directive, marking a significant evolution in privacy legislation. Its scope is broad, applying to any organization that collects or processes data of EU citizens, irrespective of the organization's geographical location. Personal data itself is defined expansively, encompassing any information relating to an identified or identifiable natural person.
A notable aspect of GDPR's influence extends beyond the European Union. Many countries worldwide have since adopted similar legislation or revised their existing data protection laws to align with GDPR principles. This global adoption suggests that GDPR has transcended its regional origins to become a de facto international benchmark for data privacy. Consequently, organizations operating on a global scale, even those outside the EU, increasingly find it strategically advantageous, if not essential, to conform to GDPR principles. This alignment helps them maintain competitiveness, build consumer trust, and navigate a converging global regulatory landscape. The widespread influence of GDPR positions it as a significant catalyst, prompting a worldwide elevation of privacy standards.
1.2 The Imperative of Data-Driven Innovation
In the contemporary digital economy, data is widely acknowledged as the "currency of progress". It is an indispensable component for training advanced AI models, driving breakthroughs in medical research, and ensuring the efficient operation of businesses. Data-driven innovation, particularly through the application of machine learning (ML) and Artificial Intelligence (AI), unlocks substantial opportunities for sophisticated analysis, accurate forecasting, and influencing human behavior. This transformative potential converts raw data and the insights derived from its processing into valuable commodities. AI systems, in many instances, can offer predictions and decisions that are not only more cost-effective but also more precise and impartial than those made by humans, by avoiding typical human psychological fallacies and being subject to rigorous controls. This continuous innovation fuels the creation of new business opportunities and provides critical insights into customer behavior, market trends, and operational efficiency.
1.3 The Inherent Tension: Privacy vs. Progress
Despite its initial acclaim as a groundbreaking legal framework, the GDPR, in its current form and application, is perceived by some as a considerable "roadblock to digital progress". Critics argue that it stifles innovation, impedes AI development, and hinders essential research. The regulation has undeniably heightened the tension between the fundamental right to privacy and the demands of the data economy.
There exists an intrinsic tension between traditional data protection principles—such as purpose limitation, data minimisation, and limitations on automated decisions—and the full, unconstrained deployment of AI and big data's capabilities. Economic literature examining the impact of GDPR enforcement has largely documented "harms to firms," including a reduction in firm performance, innovation, and competition, as well as adverse effects on web traffic and marketing activities. Specifically, venture funding for data-related technology firms has experienced a decline, and in certain instances, the GDPR has accelerated market exits and slowed new entries, particularly impacting smaller companies.
A significant observation emerging from the implementation of GDPR relates to its unintended anti-competitive effects. The high costs associated with GDPR compliance and the substantial administrative burden disproportionately affect small and medium-sized enterprises (SMEs). This often leads to market exit for some smaller firms and a reduction in new market entrants. In contrast, larger corporations, equipped with extensive financial resources (e.g., Fortune 500 firms reportedly earmarked $8 billion for GDPR upgrades), are better positioned to absorb these compliance costs. This disparity enables them to increase their market share at the expense of smaller competitors. This dynamic directly harms competition. The framework, while designed to protect individuals, may inadvertently foster an anti-competitive environment by creating a significant barrier to entry for smaller, agile innovators. This could potentially stifle overall innovation by reducing the diversity of players and ideas in the market. This situation highlights a potential need for regulatory adjustments that differentiate obligations based on factors such as firm size and the volume of data processed, as some analyses suggest.
GDPR's Foundational Principles and Their Impact on Innovation
The GDPR is structured around seven core data protection principles, outlined in Article 5: Lawfulness, Fairness, and Transparency; Purpose Limitation; Data Minimisation; Accuracy; Storage Limitation; Integrity and Confidentiality; and Accountability. These principles are foundational to understanding and adhering to GDPR requirements. While crucial for protecting individual privacy, their strict interpretation and implementation can create inherent tensions with the dynamic and exploratory nature of data-driven innovation.
2.1 Lawfulness, Fairness, and Transparency
This principle mandates that the processing of personal data must be conducted in a lawful, fair, and transparent manner. "Lawful" processing requires a valid legal basis, such as explicit consent from the data subject, contractual necessity, a legal obligation, vital interests, a public task, or legitimate interest. Organizations must meticulously document when and how consent was obtained. "Fair" processing means that the data handling should be in the best interest of the data subject, and the scope of processing should be reasonably expected by them. "Transparency" necessitates clear communication to data subjects about what data is processed, how it is processed, and why, presented in an easily understandable format, typically through comprehensive privacy policies and accessible contact information for a Data Protection Officer (DPO).
The tension with innovation arises because data-driven innovation often thrives on collecting vast amounts of data and exploring novel uses for it. The "lawful" aspect, particularly the requirement for explicit consent for each specific purpose, can significantly impede rapid experimentation and the discovery of unforeseen valuable applications for data. The "fairness" aspect can restrict the scope of data usage to only what the user "reasonably expects," which may not encompass truly novel or innovative applications that were not initially conceived. Furthermore, "transparency" demands clear and simple communication, which becomes challenging when the exact future uses of data for innovative purposes are still evolving or are inherently complex to explain to a layperson. This can lead to either overly broad consent requests that may not withstand scrutiny or overly narrow ones that inadvertently stifle innovation.
A notable challenge in this area is the phenomenon often referred to as "consent fatigue." The high standard for valid consent—requiring it to be "explicit, specific, informed, and unambiguous" —implies that organizations must frequently and meticulously request consent for various data processing activities. This constant barrage of detailed consent requests can overwhelm users, leading to a tendency to either blindly accept terms without full understanding or, in some cases, abandon services altogether. This behavioral pattern can reduce both the quantity and potentially the quality of data available for innovation. It creates a situation where the mechanism designed to empower users (consent) can inadvertently become a barrier to data collection, potentially pushing innovators towards relying on less transparent legal grounds or utilizing fully anonymized data, even if such data is less analytically rich.
2.2 Purpose Limitation
The second principle dictates that personal data should only be collected for "specified, explicit, and legitimate purposes". Critically, it should not be reused for other, incompatible purposes without obtaining new consent from the data subject or establishing a new legal basis.
This principle directly restricts a common practice in data-driven innovation: repurposing existing datasets for new insights or developing new products and services. For instance, if data was initially collected for a specific purpose, such as sending a newsletter, it cannot be readily used for an entirely different purpose, like targeted advertising based on IP addresses, without securing fresh consent or identifying a new, valid legal basis. This requirement can significantly impede or even prevent innovative projects that depend on combining and analyzing data from diverse sources for novel, unanticipated applications, thereby slowing down the pace of development.
This creates a fundamental dilemma between "dynamic data use" and "static purpose" for agile, data-driven innovation. Innovation, particularly in fields like AI and machine learning, often involves exploratory data analysis where novel insights and applications emerge after the initial data collection has occurred. However, the purpose limitation principle mandates that these purposes must be "specified, explicit, and legitimate" at the time of data collection. This forces organizations into a difficult position: they must either attempt to predict all potential future uses of data—a task often impossible for truly innovative applications—or they must repeatedly seek new consent from data subjects, which introduces significant friction into the innovation process. This dynamic can push innovation towards utilizing less personal data or developing more generalized AI models, potentially limiting the creation of highly personalized or niche solutions that could otherwise offer substantial value.
2.3 Data Minimisation
The principle of data minimisation requires organizations to collect and process only the absolute minimum amount of personal data necessary to achieve their stated purpose. This means that unnecessary data should not be indiscriminately hoarded.
This principle poses a direct tension with data-driven innovation, especially in the context of machine learning and AI. Many advanced AI models and algorithms benefit significantly from access to large and diverse datasets, as greater data volume and variety often lead to more robust and accurate models. Data minimisation, by encouraging the collection of only what is strictly necessary, can inherently limit the scope and effectiveness of such innovative projects. It can also make it more challenging to identify new patterns or correlations that might only become apparent within a broader dataset, particularly if the initial definition of "necessary" data is too narrowly conceived.
This leads to a "quality versus quantity" trade-off in AI training. While data minimization is designed to reduce privacy risks, AI models frequently require vast quantities of data to effectively learn, reduce inherent biases, and achieve optimal performance. Limiting the quantity of data collected could inadvertently impact the representativeness and generalizability of AI models, making them less accurate or more prone to specific biases if the "minimum necessary" data is not sufficiently diverse. This highlights a potential conflict between privacy by design principles, such such as data minimisation, and the technical requirements for developing high-performing advanced AI systems. Addressing this conflict may necessitate the increased adoption of privacy-enhancing technologies (PETs) like synthetic data generation or federated learning to overcome data scarcity, which in turn can add layers of complexity and cost to AI development within the EU.
2.4 Accuracy
The accuracy principle stipulates that personal data must be precise, complete, and kept up-to-date. Data controllers and processors are obligated to take "reasonable measures" to ensure this accuracy, particularly when the data's quality impacts the data subject. If data is found to be inaccurate or incomplete, it must be promptly corrected or erased.
While accurate data is universally beneficial for any data-driven initiative, the continuous requirement for "reasonable measures" to ensure accuracy can be highly resource-intensive, especially when dealing with large and dynamic datasets common in innovative projects. For certain innovative applications, such as those focused on predictive analytics or broad trend analysis, perfect real-time accuracy of every individual data point might not be the primary concern; rather, the overall statistical validity or aggregate patterns may hold more importance. The ongoing obligation to constantly update or delete inaccurate data can introduce significant operational overhead and complexity to data management processes for innovative projects that might prioritize data volume or historical trends over granular individual record precision.
2.5 Storage Limitation
This principle dictates that personal data should not be stored for any longer than is necessary to fulfill the specific purpose for which it was originally collected. Once that purpose has been achieved, the data must be securely deleted or anonymized. Organizations are required to establish clear data retention periods for different types of data.
The storage limitation principle can directly conflict with the needs of data-driven innovation, which often relies heavily on extensive historical data. Such historical data is crucial for training complex models, identifying long-term trends, or conducting retrospective analysis that can yield valuable insights. By mandating the deletion of data once its initial purpose is fulfilled, this principle can severely limit the ability of organizations to build and maintain robust historical datasets essential for future innovative projects. This often forces innovators to re-collect data, which is costly and time-consuming, or to work with smaller, time-limited datasets, thereby impeding the development of more sophisticated and accurate data-driven solutions.
This creates what can be termed the "ephemeral data" challenge for longitudinal innovation. Many advanced analytical techniques, particularly in fields such as medical research, climate science, or urban development, depend on observing changes and trends over extended periods, which inherently requires longitudinal data. The storage limitation principle, if interpreted strictly, could severely curtail such long-term research and innovation. This situation impels innovators to adopt strategies like robust anonymization or synthetic data generation for historical analysis. Alternatively, it requires them to identify and justify specific legal bases for extended data retention for research purposes, which GDPR does permit under strict conditions and with appropriate safeguards like pseudonymisation. This adds a layer of legal and technical complexity to projects that inherently require data to be maintained over extended durations.
2.6 Integrity and Confidentiality
This principle, often related to the "CIA-Triangle" (Confidentiality, Integrity, Availability), focuses on safeguarding personal data from unauthorized access, manipulation, or loss. "Integrity" ensures that personal data is accurate, complete, and cannot be altered by unauthorized individuals, thereby protecting against threats like hacking. "Confidentiality" ensures that only authorized individuals have access to and process personal data, including strict access controls within an organization. Adherence requires implementing robust technical and organizational measures, such as encryption, access controls, and regular security assessments.
Data-driven innovation frequently involves the sharing of data across diverse teams, collaboration with external partners, or the utilization of cloud-based platforms. The stringent requirements for integrity and confidentiality can erect significant barriers to such data sharing and collaboration, which are often critical for the success of many innovative projects. Implementing and maintaining robust security measures, granular access controls, and comprehensive data encryption can be complex and costly, potentially slowing down the pace at which new innovations can be developed and deployed. Balancing the essential need for data accessibility to foster innovation with the imperative for stringent security requirements presents a substantial ongoing challenge for organizations.
2.7 Accountability
The principle of accountability mandates that organizations, acting as data controllers and/or processors, must not only ensure proper data processing but also actively demonstrate their compliance with GDPR rules. This includes maintaining comprehensive documentation of how compliance is achieved, such as logging consent, conducting Data Protection Impact Assessments (DPIAs), and integrating data protection by design and by default into their systems and processes.
This principle can create tension with the fast-paced, iterative nature of data-driven innovation. The requirement for extensive documentation and demonstrable compliance can impose a significant administrative burden. When organizations are rapidly experimenting with new data uses or technologies, the continuous need to document and prove compliance can slow down the agile development process. This shifts the focus from purely technical innovation to also encompass a substantial legal and compliance overhead, which may not be easily integrated into agile development methodologies that prioritize speed and flexibility.
This leads to what can be described as a "burden of proof" on agile innovation. The accountability principle places the onus on organizations to demonstrate compliance, not merely to be compliant. This necessitates proactive documentation, regular audits, and comprehensive impact assessments. For agile innovation cycles, where rapid prototyping and iteration are key, this "burden of proof" can introduce significant friction. It requires integrating compliance checks and documentation into every stage of development, potentially slowing down the time-to-market for new data-driven products and services. Furthermore, it demands a higher level of legal and compliance expertise within innovation teams or very close collaboration with dedicated Data Protection Officers (DPOs) and legal departments.
Table 1: GDPR Principles and Their Potential Tension with Data-Driven Innovation
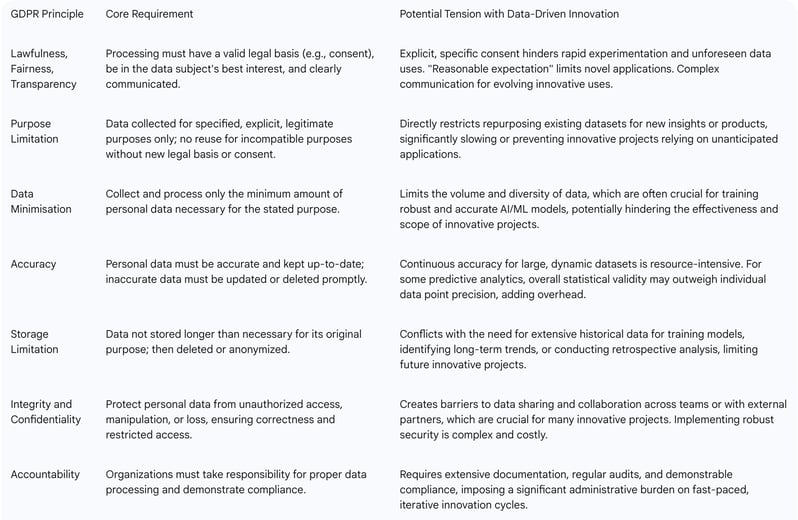
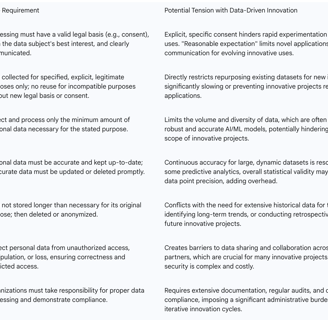
Navigating the Innovation Roadblocks: Challenges Posed by GDPR
Beyond the inherent tensions within its core principles, GDPR has presented specific, tangible hurdles and negative impacts on innovation, particularly concerning economic burdens and its effects on key technological advancements.
3.1 Economic and Operational Burdens
Economic analyses conducted since the enforcement of GDPR have largely documented adverse effects on firms, including decreased revenue, reduced profitability, and a notable decline in venture funding specifically for data-related ventures. The costs associated with GDPR compliance are substantial, with estimates suggesting an average firm of 500 employees must spend approximately $3 million to comply. This significant financial outlay has led thousands of US firms to conclude that operating in the EU is no longer worthwhile, prompting their exit from the market.
In stark contrast, large corporations such as Google, Facebook, and Amazon possess significantly larger budgets for GDPR compliance, with Fortune 500 firms reportedly earmarking $8 billion for upgrades. This financial capacity provides them with a distinct competitive advantage, enabling them to absorb compliance costs more easily and consequently increase their market share at the expense of smaller, less resourced players. Small and medium-sized enterprises (SMEs) are disproportionately burdened by these compliance requirements, exacerbated by varied national interpretations of the regulation and a general lack of resources. This confluence of factors creates a "bureaucratic bottleneck" that actively stifles economic growth and hinders research advancements across the EU.
Concrete examples illustrate this impact: companies like Williams-Sonoma, Pottery Barn, Klout (an innovative online service that closed down), Drawbridge (an identity-management company that exited the EU), Verve (a mobile marketing platform that closed its European operations), Valve, Uber Entertainment, and Gravity Interactive (game companies that shut down entire games or stopped serving EU customers due to compliance costs), and Brent Ozar Unlimited (an IT services firm) have ceased operations or exited the EU market. Furthermore, some US media outlets have resorted to blocking EU traffic to avoid compliance complexities.
This situation highlights an unintended anti-competitive effect of GDPR. The high compliance costs and administrative burden disproportionately affect SMEs, leading to their market exit and a reduction in new market entrants. Simultaneously, large firms, with their vast resources, can absorb these costs, leading to increased market concentration. This dynamic directly harms competition. The framework, while aiming to protect individuals, has created an economic moat for tech giants, potentially stifling the very innovation it seeks to foster by reducing the diversity of players and ideas in the market. This suggests a compelling need for regulatory adjustments that differentiate obligations based on firm size and the volume of data processed, as some analyses have proposed.
3.2 Impact on Specific Technologies
The broad scope of GDPR has significant implications for various cutting-edge technologies, particularly those heavily reliant on data processing.
AI and Big Data
Artificial Intelligence (AI) is not explicitly mentioned in the GDPR, yet many of its provisions are highly relevant to AI and are challenged by the novel ways personal data is processed through AI systems. There is an inherent tension between traditional data protection principles—such as purpose limitation, data minimisation, and limitations on automated decisions—and the full deployment of AI and big data's capabilities. AI models require large and diverse datasets for effective training, a requirement that current GDPR provisions (especially data minimisation and purpose limitation) make highly challenging within Europe, often compelling companies to seek alternative development environments outside the EU. GDPR also mandates explicit consent for the use of personal data by AI models, requiring that such consent be willingly provided, specific, informed, and unequivocal.
Article 22 of GDPR grants data subjects the right not to be subjected to decisions based solely on automated processing, including profiling, if these decisions produce legal effects concerning them or similarly significantly affect them. This provision impacts AI systems that involve profiling and automated decision-making, necessitating specific exceptions (e.g., contractual necessity, legal authorization, or explicit consent) and often requiring human intervention or explanations for AI-driven decisions. Furthermore, the processing of "special categories of personal data" (e.g., health, genetic, biometric data) is generally prohibited under Article 9, unless stringent exceptions apply, such as explicit consent, substantial public interest (backed by Union or Member State law), healthcare purposes, or for archiving, scientific, or historical research with robust safeguards like pseudonymisation. This significantly impacts AI development in highly sensitive sectors like healthcare.
A key challenge in AI is the "black box" problem, where many advanced AI systems operate opaquely, making their decision-making processes difficult to understand. GDPR's transparency principle and Article 22's "right to explanation" for automated decisions directly challenge this opacity. This means that AI innovation cannot solely focus on maximizing predictive power; it must also prioritize explainability and interpretability, which can be technically complex and costly to implement. This implies a necessary shift towards "Explainable AI" (XAI) as a compliance imperative, potentially slowing down development or limiting the adoption of certain opaque AI models within the EU.
IoT (Internet of Things)
Internet of Things (IoT) devices frequently collect personal data, making GDPR highly relevant to their design and operation. Key challenges include obtaining explicit consent for data processing, particularly for minors, as many IoT devices inherently lack traditional user interfaces for displaying consent forms. The very essence of IoT relies on machine-to-machine communication without direct human intermediation, which complicates the process of achieving granular consent and maintaining user control over data flow. Moreover, automated decision-making without explicit consent is prohibited if it produces "significant effects" on an individual, a core feature of many IoT applications. Due to the use of new technologies and the high risk posed to data subjects' rights and freedoms, Data Protection Impact Assessments (DPIAs) are almost always mandatory for IoT projects.
Blockchain
Blockchain technology's inherent immutability fundamentally conflicts with GDPR's "right to erasure" (also known as the Right to be Forgotten). GDPR mandates that personal data must be easily retrievable and deletable upon request. This creates significant legal and compliance challenges for organizations leveraging blockchain for data integrity, potentially increasing operational costs and reputational risks if these conflicts are not proactively addressed.
This situation represents a "immutability versus erasure" paradigm clash. Blockchain's core value proposition revolves around immutable, tamper-proof records. Conversely, GDPR's Right to Erasure (Article 17) grants individuals the right to have their personal data deleted. These two foundational principles are inherently at odds. This highlights a significant legal and technical challenge for blockchain-based innovations involving personal data. It suggests that pure on-chain storage of personal data is problematic under GDPR, pushing solutions towards hybrid models (e.g., off-chain storage for personal data with on-chain hashes) or advanced anonymization techniques. This effectively limits the scope of certain blockchain applications or necessitates complex architectural workarounds, potentially hindering the full potential of decentralized data systems in the EU.
3.3 Regulatory Ambiguity and Fragmented Enforcement
The GDPR's extensive scope, combined with its "vague clauses" and "open standards," has resulted in considerable ambiguities and challenges in interpretation. This lack of clear, prescriptive guidelines makes it difficult for businesses to effectively navigate and implement the regulation.
Further complicating matters is the fragmented enforcement across EU Member States, where each jurisdiction may apply its own interpretation of the rules. This inconsistency creates a "lack of legal certainty" for businesses and researchers operating across borders. Such regulatory fragmentation translates into substantial compliance costs and administrative hurdles, which are particularly burdensome for startups and SMEs that lack the resources to navigate 27 different legal landscapes.
This situation imposes what can be termed an "uncertainty tax" on innovation. The ambiguity in legal interpretation and the fragmented enforcement across Member States create an environment of legal uncertainty for businesses. This uncertainty acts as a "tax" on innovation, as firms must either over-comply to mitigate perceived risks or face potential fines and legal challenges. This can deter investment in new data-driven ventures within the EU or encourage companies to seek out less stringent jurisdictions. It implies that while the GDPR aims to foster a unified digital market, its practical application can inadvertently create internal market barriers, thereby hindering the EU's ambition to be a digital leader. Therefore, harmonization of enforcement and the provision of clearer, more consistent guidance are critical steps towards fostering a predictable and conducive environment for innovation.
4. Unlocking Innovation: Opportunities and Enablers within GDPR
While GDPR presents significant challenges, it also fosters innovation by building trust, enhancing data quality, and providing strategic advantages. The regulation, despite its strictures, can be viewed as a framework that encourages a more responsible and sustainable approach to data-driven progress.
4.1 Building Trust as a Catalyst for Data Sharing
Privacy is increasingly recognized as a key enabler of innovation, fundamentally because consumer trust is central to the success of businesses offering data-driven products and services. The GDPR significantly strengthens individuals' data protection rights, empowering them with greater control over their information, including the right to access, rectify, and erase their data. This enhanced transparency regarding data processing practices fosters a deeper level of trust between businesses and their customers.
When individuals are confident that their personal data is being handled responsibly and ethically, they are demonstrably more likely to engage with businesses and willingly share their information. This increased trust, in turn, can lead to more willing consent for data processing activities. This dynamic suggests a positive feedback loop: stronger privacy protections lead to greater consumer trust, which then translates into a more willing and potentially higher-quality "supply" of personal data from consumers. For innovative businesses, investing in GDPR compliance is not merely a regulatory cost but a strategic investment in building a sustainable and ethically sourced data pipeline. This approach can yield more valuable datasets in the long run and serve as a powerful differentiator against competitors who may not prioritize data protection to the same extent.
4.2 Enhanced Data Quality and Security
The GDPR mandates that organizations implement robust security measures, thereby fostering a comprehensive data protection culture and significantly reducing the risk of data breaches. This includes the regular assessment and updating of security protocols, the implementation of strong encryption, and the establishment of stringent access controls.
A less frequently discussed, yet significant, benefit of the GDPR is the resulting improvement in overall data quality. The process of cleaning up and organizing data to achieve GDPR compliance often leads to increased data accuracy and consistency. Furthermore, streamlining data management processes as mandated by GDPR can also lead to reduced operational costs and improved efficiency in data analysis. This indicates that GDPR, while imposing initial costs, acts as a powerful catalyst for improved data hygiene and governance. Cleaner, more accurate, and better-secured data is inherently more valuable for any data-driven innovation, as it leads to more reliable insights and the development of more effective AI models. In this context, the regulatory burden transforms into an operational advantage, fundamentally improving the quality of data assets available for innovation.
4.3 Strategic Competitive Advantage
Compliance with GDPR offers tangible strategic benefits beyond mere legal adherence. It helps businesses avoid significant penalties, which can severely impact a company's reputation and financial stability. Furthermore, obtaining a GDPR certification or consistently demonstrating strong compliance can serve as a distinct competitive advantage in the marketplace, signaling a profound commitment to data protection and privacy to both customers and partners.
The global influence of GDPR also contributes to a more harmonized approach to data protection worldwide, fostering a consistent standard for privacy rights. For businesses that are compliant, this global alignment can simplify international operations, reducing the complexities associated with cross-border data transfers and varying national regulations. This shifts the perception of privacy from a "cost center" to a "value driver." It encourages companies to innovate not only in terms of data utility but also in developing privacy-enhancing features and transparent data practices. This can lead to the creation of new market segments for "privacy-friendly" products and services, representing a significant strategic opportunity for businesses to gain a competitive edge in the evolving digital economy.
4.4 Fostering Ethical Data Practices
The GDPR actively encourages the cultivation of a culture of accountability and transparency within organizations concerning their data handling practices. A cornerstone of this ethical approach is the promotion of "Privacy by Design and by Default," which advocates for the integration of privacy measures directly into the architecture of systems and operations from the very outset of their development.
This aligns seamlessly with broader ethical considerations in AI and data processing, such as ensuring genuinely informed consent, maintaining transparency about how data is used, and implementing robust protections against unauthorized access. This emphasis on ethical principles implies that innovation under GDPR is not solely about what is legally permissible, but also about what is ethically responsible. This fosters a more sustainable model of innovation, significantly reducing the risk of public backlash, regulatory intervention, and reputational damage that can arise from questionable or unethical data practices. It positions ethical considerations as an integral component of long-term business viability and crucial for societal acceptance of new technologies.
5. Strategic Frameworks and Best Practices for Harmonization
To effectively balance the imperative of data protection with the demands of innovation, organizations can adopt several strategic frameworks and best practices. These approaches provide concrete pathways to achieve compliance while simultaneously fostering growth and technological advancement.
5.1 Privacy by Design and by Default
This fundamental principle mandates that data protection and privacy considerations must be built into the design of processing operations and information systems from their inception, rather than being treated as an afterthought. "Privacy by Default" further specifies that service settings must automatically be data protection-friendly, ensuring that the most privacy-protective options are the default choices for users.
When correctly designed and implemented, Privacy by Design does not hinder the development of AI systems, even if it entails some additional initial costs. It ensures that privacy considerations are deeply embedded into the foundational architecture of digital identity frameworks and other data-driven systems. This proactive approach encourages innovation by mitigating the fear of costly rectifications or public backlash later in the development cycle, as privacy is integrated from the very beginning.
This approach represents a significant shift from viewing privacy as a compliance burden to recognizing it as a core architectural principle. While Privacy by Design is often initially perceived as an additional cost or hurdle , by embedding privacy from the earliest design stages, it transforms from a mere compliance checkbox into a fundamental architectural principle for data systems and products. This shifts the innovation mindset from "how to get around GDPR" to "how to build inherently privacy-preserving innovation." It encourages the development of technologies and systems that are designed to protect data from the ground up, which can be a significant competitive advantage and reduce long-term legal and reputational risks. Privacy by Design thus becomes a guiding framework for responsible innovation, influencing core technical choices and fostering trust.
5.2 Leveraging Pseudonymisation and Anonymisation
These two techniques offer distinct yet complementary methods for managing data privacy while enabling data utility.
Pseudonymisation refers to techniques that replace identifiable information within a dataset with pseudonyms, with the original identifying information kept separately and securely. Crucially, pseudonymised data remains personal data under GDPR, but the risk to data subjects is significantly reduced because direct identification is no longer possible without the additional, separately held information.
Anonymisation, in contrast, involves transforming data in such a way that individuals are no longer identifiable, even with additional information. Truly anonymised data falls outside the scope of GDPR, meaning it is not subject to the same stringent restrictions as personal data. Achieving this requires robust methods to prevent re-identification, including the secure deletion of original identifying information.
Both techniques offer significant innovation benefits. Pseudonymisation reduces privacy risks, aids in implementing data protection by design, enhances security, and, critically, supports the re-use of personal data for new purposes. This is particularly relevant for archiving, scientific or historical research, statistical purposes, other compatible purposes, and general analysis. It optimizes the inherent trade-offs between data utility and privacy by allowing for richer analysis than full anonymisation while still providing substantial safeguards.
Anonymisation, by removing legal constraints (as the data is no longer "personal"), offers maximum freedom for its use and facilitates wider sharing for research and development purposes without the need for complex data sharing agreements or ongoing privacy concerns. Both pseudonymisation and anonymisation are crucial for AI/ML development, enabling organizations to derive valuable insights from large datasets while safeguarding individual privacy.
The clear distinction between pseudonymisation (where data remains personal but risks are reduced) and anonymisation (where data loses its personal status, offering higher utility freedom) creates a spectrum of data utility and privacy risk management options. This implies that innovators have a flexible toolkit to manage privacy risks based on their specific needs. For highly sensitive or broad-purpose innovation, full anonymization offers regulatory freedom. For applications requiring some level of re-identifiability or richer data, pseudonymization allows for greater utility while providing significant privacy safeguards. This encourages a risk-based approach to data handling, where the chosen technique aligns with the innovation's purpose and sensitivity, thereby optimizing the balance between utility and privacy.
5.3 The Role of Data Protection Impact Assessments (DPIAs)
Data Protection Impact Assessments (DPIAs) are systematic processes designed to analyze personal data processing activities, identify potential data protection risks, and implement measures to mitigate those risks. Under Article 35 of GDPR, DPIAs are legally required when processing is likely to result in a "high risk" to data subjects' rights and freedoms, especially when new technologies are being used, large-scale processing activities are undertaken, or systematic monitoring is involved.
DPIAs are more than just a compliance exercise; they serve as a proactive tool. They help organizations prioritize risks, manage them proportionately, and resolve potential problems at an early stage of a project. Consistent use of DPIAs increases awareness of privacy issues throughout an organization, fostering a 'data protection by design' approach from the outset. Through the DPIA process, organizations can also uncover opportunities to improve their data privacy practices, enhance accountability, and strengthen their overall data governance, leading to more robust and trustworthy data processing. By providing a structured approach for assessing the impact of data processing, DPIAs enable organizations to confidently explore new data processing methods or technologies, as they have a clear framework to assess and manage associated risks.
DPIAs can be seen as an "innovation de-risking" mechanism. DPIAs are mandatory for high-risk processing and the deployment of new technologies. While often perceived as an administrative burden, they compel a proactive assessment of privacy risks before the deployment of new data-driven initiatives. This transforms the DPIA into a critical "innovation de-risking" tool. By identifying and mitigating potential risks early in the development lifecycle, organizations can avoid costly legal battles, reputational damage, or the need to abandon innovative projects post-launch. This shifts the perception of a compliance requirement into a strategic planning tool that ensures innovations are built on a sound privacy foundation, significantly increasing their chances of successful and sustainable deployment.
5.4 Robust Data Governance Frameworks and Compliance Tools
A robust data governance framework provides an agreed-upon system that assigns ownership, standardizes processes, and embeds controls to ensure data is trustworthy and compliant. Such a framework typically includes comprehensive policies, procedures, and controls for data collection, storage, access, and deletion.
Effective data governance directly supports compliance with GDPR. Modern frameworks, built on foundational pillars of people, process, technology, and policy, move beyond traditional, heavy documentation. Instead, they create operational guardrails that empower teams to deliver trusted data at scale, thereby fostering both innovation and compliance simultaneously. Furthermore, specialized GDPR compliance tools enhance data security, streamline data management processes, improve customer trust, and facilitate complex international data transfers. These tools can automate repetitive privacy tasks, provide continuous monitoring capabilities, and offer decision-support guidance for incident response, significantly improving operational efficiency and risk management.
Robust data governance serves as the foundation for scalable innovation. GDPR demands stringent accountability and structured data handling. Data governance frameworks provide this necessary structure, evolving from static policies to dynamic, automated systems. This implies that robust data governance is not merely about compliance, but about building the fundamental operational infrastructure necessary for
scalable data-driven innovation. By ensuring data quality, clear lineage, appropriate access controls, and consistent policy enforcement, effective governance enables organizations to confidently expand their data initiatives, integrate new technologies, and manage complex data flows without compromising privacy or incurring unforeseen risks. It serves as the operational backbone that allows innovation to flourish responsibly and sustainably.
5.5 Navigating Legal Bases for Processing
GDPR Article 6 outlines six lawful bases for processing personal data: consent, contractual necessity, compliance with legal obligations, vital interests, public interest/official authority, and legitimate interests. Organizations are required to identify the appropriate legal basis in advance of any processing activity and ensure thorough documentation to justify their choice.
Each legal basis has distinct implications for innovation:
Consent: Requires explicit, specific, informed, and unambiguous agreement from the data subject, and must be easily withdrawable. While a primary basis, its specificity can be challenging for dynamic and evolving innovation, as it may necessitate repeated consent requests for new or unforeseen uses.
Legitimate Interests: This basis requires a careful balancing act between the organization's interests and the data subject's rights and interests. The European Data Protection Board (EDPB) provides a three-step test for relying on legitimate interests: the interest must be lawful, clearly and precisely articulated, and real and present (not speculative). It can be an appropriate legal basis for AI model training and development under specific conditions, provided a thorough impact assessment is conducted to demonstrate that the organization's interests are not overridden by the data subject's fundamental rights.
Public Interest/Scientific Research: GDPR allows for processing for statistical, scientific, or historical research purposes, often with appropriate safeguards such as pseudonymisation, which can provide a valid basis for extensive data use in these domains.
The strategic choice of legal basis is critical for AI development. While consent is often the most obvious legal basis, other grounds like "legitimate interests" or "public interest/scientific research" offer greater flexibility for AI development, especially when dealing with large, diverse datasets or purposes that evolve over time. This implies that legal counsel and AI developers must strategically choose the most appropriate legal basis, moving beyond a default reliance on consent. Leveraging legitimate interests or public interest for AI training, when applicable and accompanied by proper safeguards and DPIAs, can significantly reduce the administrative burden and enable more extensive data processing for innovative purposes, particularly where obtaining explicit consent for every unforeseen use is impractical.
5.6 Addressing Automated Decision-Making (Article 22) and Special Categories of Data (Article 9)
These two articles impose specific restrictions that directly impact advanced data-driven innovations, particularly in AI.
Automated Decision-Making (Article 22): Data subjects have the right not to be subject to a decision based solely on automated processing (including profiling) which produces legal effects concerning them or similarly significantly affects them. Exceptions apply if the decision is necessary for entering into or performing a contract, is authorized by Union or Member State law (with suitable safeguards), or is based on the data subject's explicit consent. Crucial safeguards include the right to obtain human intervention, to express one's point of view, and to contest the automated decision.
Special Categories of Data (Article 9): This article establishes a general prohibition on processing sensitive personal data, which includes data revealing racial or ethnic origin, political opinions, religious or philosophical beliefs, or trade union membership, as well as genetic data, biometric data for unique identification, data concerning health, or data concerning a natural person's sex life or sexual orientation. Processing of these categories is only permitted under specific, stringent exceptions, such as explicit consent, vital interests, reasons of substantial public interest (based on Union or Member State law), healthcare purposes, or for archiving, scientific, historical research, or statistical purposes, provided appropriate safeguards are in place.
These articles directly impact AI applications in sensitive domains like finance (e.g., credit scoring), healthcare (e.g., medical diagnosis), employment, and education, where automated decisions or sensitive data are frequently utilized. Compliance requires careful design of AI systems to allow for human oversight, ensure transparency in decision-making processes, and implement robust safeguards (e.g., encryption, pseudonymisation) for sensitive data.
5.7 Guidance from Regulatory Bodies (e.g., EDPB, CNIL)
Regulatory bodies such as the European Data Protection Board (EDPB) and national authorities, like the CNIL in France, play a crucial role in clarifying GDPR's application. They regularly issue guidelines, recommendations, and opinions, particularly addressing new and complex technologies like AI.
This ongoing guidance provides much-needed legal certainty for businesses, fostering AI innovation while ensuring the protection of fundamental rights. For example, the CNIL has suggested a flexible interpretation of purpose limitation for general-purpose AI systems, allowing operators to describe the type of system and its key functionalities rather than defining all potential applications at the training stage. They have also justified extended data retention for valuable research datasets, provided appropriate security measures are in place. The EDPB's Opinion 28/2024 specifically clarifies the assessment of anonymity for AI models and outlines the conditions for relying on legitimate interests as a legal basis for AI development and deployment.
The evolving regulatory dialogue serves as an important enabler for innovation. GDPR's "vague clauses and open standards" and the rapid pace of technological innovation mean that static legislation alone is insufficient. Regulatory bodies actively engage in issuing guidance and opinions, particularly for AI. This ongoing dialogue and evolving guidance are critical for fostering responsible innovation. It means that while initial legal texts might be broad, regulators are working to provide practical interpretations that allow for the development and deployment of new technologies. Companies that actively monitor and engage with this guidance can proactively adapt their strategies, reducing uncertainty and finding compliant pathways for cutting-edge technologies. This highlights the importance of legal foresight and continuous learning for innovators.
5.8 Industry Codes of Conduct and Certifications
GDPR explicitly encourages industry associations to develop codes of conduct that specify the application of the regulation, taking into account sector-specific features and the particular needs of micro, small, and medium-sized enterprises (SMEs). Additionally, GDPR certifications are voluntary processes where organizations undergo assessment by accredited bodies to verify their compliance with GDPR requirements.
These mechanisms offer significant innovation benefits. Codes of conduct provide practical, defensible frameworks that improve consistency in data processing, strengthen compliance, and support internal or partner alignment, especially for complex cross-entity data sharing scenarios. Certifications, on the other hand, demonstrably signal an organization's commitment to data protection and privacy, which can significantly increase customer trust, reduce the risk of data breaches, and provide a tangible competitive advantage in the marketplace. By adhering to industry-specific best practices and obtaining certifications, businesses can streamline their compliance efforts, build a stronger reputation, and foster greater confidence among their data subjects, ultimately creating a more conducive environment for responsible innovation.
Conclusion
Balancing data protection and innovation under GDPR is not a zero-sum game, but rather a complex, evolving challenge that demands strategic foresight and adaptive implementation. While the regulation's stringent principles—such as purpose limitation, data minimisation, and accountability—initially presented significant hurdles, particularly for smaller firms facing substantial compliance costs and fragmented enforcement, a deeper analysis reveals opportunities for synergistic growth.
The initial economic impacts, including reduced venture funding and market concentration favoring larger entities, underscore the need for a nuanced regulatory approach that considers firm size and technological specificities. However, the very mechanisms of GDPR, by prioritizing transparency and accountability, foster a foundation of trust that is indispensable for the long-term sustainability of data-driven economies. When individuals are confident their data is protected, they are more willing to share it, thereby enriching the datasets available for innovation.
Furthermore, the compliance journey itself often compels organizations to improve data quality, enhance security measures, and establish robust data governance frameworks. These are not merely regulatory burdens but strategic investments that yield cleaner, more reliable data, which is inherently more valuable for developing sophisticated AI models and other data-driven solutions. Frameworks like Privacy by Design, coupled with the strategic use of pseudonymisation and anonymisation, offer practical pathways to embed privacy into the core of innovation, transforming compliance into an architectural principle rather than an afterthought.
The ongoing guidance from regulatory bodies like the EDPB and CNIL is crucial in navigating the ambiguities of GDPR concerning emerging technologies like AI, IoT, and blockchain. This dynamic regulatory dialogue provides much-needed clarity, allowing innovators to adapt their strategies and explore new frontiers responsibly. Ultimately, the GDPR pushes organizations towards ethical data practices, recognizing that responsible innovation, built on trust and strong data hygiene, is not just a legal obligation but a powerful competitive differentiator and a long-term sustainability strategy in the digital age. The future of data-driven innovation within the EU will depend on a continuous, collaborative effort between regulators, industry, and technologists to refine interpretations, develop practical solutions, and foster an ecosystem where privacy and progress can genuinely co-exist and reinforce each other.