Navigating GDPR Compliance in the AI Era
Explore how GDPR regulations impact AI and machine learning development, implementation, and operations with practical compliance strategies for businesses in 2025.
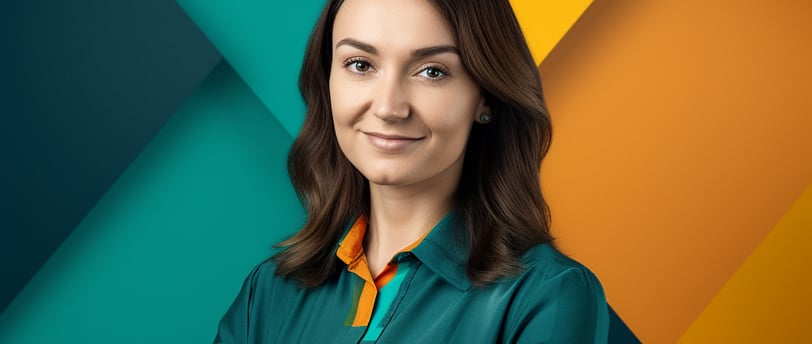
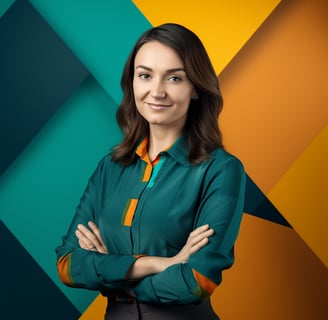
In an era where data drives innovation, artificial intelligence stands at a crossroads with data protection regulations. Since its implementation in 2018, the General Data Protection Regulation (GDPR) has fundamentally transformed how organizations approach AI development and deployment—creating a complex dance between technological advancement and individual privacy rights. Organizations now find themselves navigating an intricate landscape where the insatiable appetite of machine learning algorithms for data directly conflicts with regulatory principles designed to limit data collection, processing, and retention. This tension is particularly evident as we look at the state of AI in 2025, where sophisticated neural networks and predictive algorithms have become integral to business operations across sectors. Far from being merely a compliance hurdle, the relationship between GDPR and AI represents one of the most significant challenges in modern technology governance, affecting everything from how algorithms are designed to the ways in which businesses deliver personalized services. This article explores the multifaceted impact of GDPR on artificial intelligence and machine learning, offering practical compliance strategies, examining real-world implementation challenges, and providing a roadmap for organizations seeking to innovate responsibly in this regulated environment.
Understanding GDPR Principles in the Context of AI
Core GDPR Principles Affecting AI Development
The foundational principles of GDPR present specific challenges for AI systems that organizations must carefully address to maintain compliance. Data minimization, a cornerstone of the regulation, requires organizations to collect only data that is necessary for specified purposes—a principle that often contradicts the traditional machine learning approach of gathering vast amounts of information to improve model accuracy. Purpose limitation similarly restricts organizations from repurposing collected data for AI applications that weren't initially specified, creating roadblocks for teams wanting to leverage existing datasets for new machine learning initiatives. Storage limitation adds another layer of complexity by requiring organizations to delete data when it's no longer needed, directly conflicting with the common practice of maintaining historical training data indefinitely to refine algorithms over time. These principles collectively force organizations to adopt more disciplined approaches to data collection and AI development, moving away from opportunistic data gathering toward strategically planned acquisition tied to specific, documented objectives. Leading organizations have transformed these constraints into competitive advantages by designing more efficient algorithms that require less data and implementing sophisticated data governance frameworks that ensure compliance while still enabling innovation.
The GDPR's emphasis on individual rights creates additional considerations for AI systems processing personal data. The right to erasure ("right to be forgotten") poses particular challenges for machine learning models, as removing an individual's data from training sets can affect model performance and potentially require retraining entire systems. The right to object to processing, including profiling and automated decision-making, means organizations must build mechanisms for humans to review significant AI-driven decisions when requested. Perhaps most challenging is the right to explanation, derived from transparency requirements in Articles 13-15, which implies individuals should receive meaningful information about the logic involved in automated decisions affecting them. This right directly challenges the "black box" nature of many advanced machine learning algorithms, particularly deep learning systems whose internal operations can be difficult to interpret even for their creators. Organizations implementing AI must therefore carefully evaluate the explainability of their algorithms, potentially making trade-offs between performance and transparency based on the context and impact of their applications. These individual rights collectively push AI development toward more human-centered approaches that respect personal autonomy and provide mechanisms for redress when automated systems make consequential decisions.
Defining Automated Decision-Making Under GDPR
Article 22 of GDPR addresses automated decision-making and profiling, creating specific restrictions that have profound implications for AI applications. This provision gives individuals the right not to be subject to purely automated decisions that produce legal or similarly significant effects, with limited exceptions for contractual necessity, legal authorization, or explicit consent. When organizations rely on these exceptions, they must implement appropriate safeguards, including the right to obtain human intervention, express one's point of view, and contest the decision. The practical application of Article 22 has proven challenging for organizations deploying AI systems, particularly in determining what constitutes a "significant effect" on individuals. Financial services firms have generally accepted that credit decisions fall within this scope, while retailers debate whether personalized pricing algorithms meet the threshold. Healthcare organizations must carefully navigate automated diagnostic tools, and recruitment platforms face scrutiny over automated candidate screening. In response to these requirements, many organizations have implemented "human-in-the-loop" approaches where automated systems make recommendations rather than final decisions, though regulators increasingly scrutinize whether such human oversight is meaningful or merely perfunctory. The European Data Protection Board's guidance suggests that nominal human involvement does not exempt a process from Article 22 if the human does not meaningfully influence the outcome.
The concept of profiling under GDPR has particular relevance for machine learning systems that categorize or make predictions about individuals. Article 4 defines profiling as "any form of automated processing of personal data consisting of the use of personal data to evaluate certain personal aspects relating to a natural person," specifically mentioning work performance, economic situation, health, personal preferences, interests, reliability, behavior, location, and movements. This broad definition encompasses many common applications of machine learning, from recommendation systems to predictive analytics tools that assess customer behavior or employee performance. When profiling is combined with automated decision-making that produces significant effects, the full restrictions of Article 22 apply. Even when profiling falls short of this threshold, organizations must still provide specific information to data subjects under Articles 13 and 14, including meaningful information about the logic involved and the significance and envisaged consequences of such processing. Organizations must therefore carefully assess whether their AI applications constitute profiling under GDPR and implement appropriate notices, consent mechanisms, and safeguards based on this determination. This assessment becomes particularly complex for advanced AI systems that may discover unexpected correlations or infer sensitive attributes from seemingly innocuous data, requiring ongoing monitoring and assessment rather than one-time compliance checks.
Key Compliance Challenges for AI Systems
Explainability and Transparency Requirements
The "black box" nature of many advanced machine learning models creates significant tensions with GDPR's transparency requirements. Neural networks, particularly deep learning systems, often operate through complex layers of mathematical calculations that transform inputs into outputs without producing humanly understandable explanations for specific decisions. This opacity directly conflicts with the GDPR's requirement that data subjects receive "meaningful information about the logic involved" in automated processing. The struggle to reconcile these competing realities has driven substantial investment in "explainable AI" (XAI) approaches that aim to make machine learning models more interpretable without sacrificing performance. Some organizations address this challenge by using inherently more transparent algorithms such as decision trees or rule-based systems for high-risk applications, even when more complex models might offer marginally better performance. Others employ post-hoc explanation techniques like LIME (Local Interpretable Model-agnostic Explanations) or SHAP (SHapley Additive exPlanations) values that help identify which features most influenced particular predictions. The appropriate approach to explanation depends heavily on the context, with higher-risk applications warranting more comprehensive explanations and greater investments in algorithmic transparency.
Organizations must also balance intellectual property considerations with transparency requirements when explaining their AI systems. While algorithm details may represent valuable trade secrets, regulators have increasingly made clear that commercial interests cannot override individuals' fundamental rights to explanation for decisions that significantly affect them. This tension has led many organizations to develop tiered explanation approaches that provide different levels of detail to different stakeholders: general methodology explanations for all data subjects, more detailed information upon specific request, and comprehensive technical documentation for supervisory authorities when required. Many financial institutions, for example, have developed standardized explanation frameworks for credit decisions that explain key factors influencing outcomes without revealing proprietary model details. Healthcare organizations similarly provide patients with understandable explanations of diagnostic algorithms while maintaining more detailed technical documentation for regulatory review. The development of industry standards and best practices for AI transparency continues to evolve, with organizations that take proactive approaches to explanation often gaining competitive advantages through enhanced customer trust and reduced regulatory risk. As the EDPB and national data protection authorities issue more specific guidance on explanation requirements, organizations should remain flexible in their approaches while maintaining core commitments to meaningful transparency.
Training Data Compliance Issues
The enormous appetite of machine learning systems for training data creates substantial GDPR compliance challenges, particularly regarding establishing appropriate legal bases for processing. While consent serves as a commonly cited basis, obtaining valid GDPR-compliant consent for AI training purposes can be problematic, especially for large-scale data collection or when the specific AI applications weren't envisioned at collection time. Legitimate interest offers an alternative basis but requires organizations to document a thorough balancing test weighing their interest in AI development against potential privacy impacts on individuals. Contractual necessity applies in limited circumstances where AI is essential to delivering services explicitly requested by users. Organizations often implement a combination of these bases depending on context, with sophisticated governance frameworks determining which data can be used for which AI applications based on how it was originally collected. When acquiring third-party data for AI training, due diligence becomes essential to verify that proper consent or other legal bases were established and that these extend to the intended AI use cases. Many organizations now incorporate specific provisions about AI training in their data processing agreements and conduct regular audits of training data provenance to ensure compliance throughout the model development lifecycle.
Special category data presents heightened challenges for AI systems, as GDPR imposes stricter requirements on processing information related to race, ethnicity, political opinions, religious beliefs, health, sexual orientation, and biometric data. Such sensitive data often proves valuable for machine learning applications in healthcare, human resources, and financial services, creating significant compliance hurdles. Organizations must identify not only a general legal basis under Article 6 but also satisfy one of the specific conditions in Article 9, such as explicit consent or substantial public interest. The challenge becomes particularly acute when AI systems might inadvertently infer special category data from seemingly innocuous inputs—for example, deriving health information from shopping patterns or identifying religious affiliation from behavioral data. Organizations must conduct thorough data classification to identify special category data in training sets and implement appropriate safeguards such as enhanced consent mechanisms, anonymization techniques, or restricted processing environments. Beyond strict compliance considerations, the use of special category data in AI raises important ethical questions about discrimination and bias, as protected characteristics may influence algorithmic outcomes even when not explicitly included as model features. Leading organizations implement comprehensive bias detection and mitigation frameworks that regularly test models for disparate impact across protected groups and adjust algorithms to reduce unfair outcomes.
Cross-Border Data Transfers for Global AI Operations
The global nature of AI development creates significant challenges under GDPR's restrictions on international data transfers. Many organizations maintain centralized AI research teams or use cloud-based machine learning platforms operated from countries without adequacy decisions, particularly the United States. Following the Schrems II decision invalidating the EU-US Privacy Shield and imposing additional requirements for Standard Contractual Clauses (SCCs), organizations face increased scrutiny when transferring European personal data to such countries. This has profound implications for AI operations, requiring organizations to implement comprehensive transfer impact assessments evaluating whether recipient countries offer sufficient protection against government access to personal data. These assessments have proven challenging for transfers to major AI development hubs, leading many organizations to adopt multi-faceted compliance strategies. Some have regionalized their AI operations, establishing separate European development environments with data localization measures to avoid transfers entirely. Others have implemented technical safeguards like advanced encryption, pseudonymization, or federated learning approaches that allow model development without transferring raw personal data. Many have renegotiated contracts with cloud providers to include enhanced safeguards against foreign government access, though questions remain about the efficacy of purely contractual measures.
The regulatory landscape for international data transfers continues to evolve, creating ongoing compliance challenges for global AI initiatives. The EU-US Data Privacy Framework provides a new mechanism for certain transfers to the United States, though many organizations remain cautious given the history of previous frameworks being invalidated. The revised Standard Contractual Clauses adopted in 2021 offer more flexible options for different transfer scenarios but require significant implementation effort including data mapping, gap analysis, and development of supplementary measures where necessary. Beyond European requirements, organizations must navigate an increasingly complex global patchwork of data localization and transfer restrictions, with countries like China, Russia, India, and Brazil implementing their own requirements that sometimes conflict with GDPR approaches. This fragmented landscape has driven some organizations to develop regionally segregated AI infrastructures, while others implement sophisticated data governance frameworks that route data and model training activities through compliant pathways based on data origin and classification. Striking the right balance between global AI capabilities and regional compliance requirements remains one of the most challenging aspects of GDPR implementation for multinational organizations, requiring close collaboration between technical, legal, and business stakeholders to develop sustainable approaches that enable innovation while managing regulatory risk.
Practical Compliance Strategies
Privacy by Design in AI Development
Privacy by Design has evolved from a theoretical concept to a practical requirement under GDPR, with particular relevance for AI development. This approach requires embedding privacy considerations throughout the entire AI lifecycle rather than treating them as an afterthought or compliance checkbox. In practice, this means involving privacy experts during the initial conception of AI projects, incorporating privacy requirements into technical specifications, and establishing privacy checkpoints at key development milestones. Organizations implementing Privacy by Design typically begin with a clear data strategy that defines what personal data will be used for which AI applications and under what conditions. This strategy guides important decisions such as whether to use synthetic data instead of real personal data for initial development, which data elements should be excluded from models despite their potential predictive value, and what privacy-enhancing technologies should be applied to different datasets. Leading organizations have developed AI-specific privacy impact assessment templates that help development teams systematically identify and address privacy risks before significant resources are invested. This preventative approach not only supports compliance but often leads to more thoughtful system design that better respects individual privacy while still achieving business objectives. When implemented effectively, Privacy by Design creates a culture where privacy becomes an integral part of AI innovation rather than a constraint imposed upon it.
The concept of data protection impact assessments (DPIAs) plays a crucial role in Privacy by Design for AI systems. GDPR requires DPIAs when processing is likely to result in high risk to individuals' rights and freedoms, a threshold that many AI applications meet, particularly those involving profiling, large-scale processing of special category data, or systematic monitoring of publicly accessible areas. A well-executed DPIA for AI systems typically involves a multidisciplinary team assessing factors such as the necessity and proportionality of processing, risks to individuals, and measures to address those risks. For AI applications, this assessment should cover algorithm selection, training data composition, accuracy metrics, potential biases, security measures, and procedures for handling data subject requests. The most effective DPIAs don't merely document existing designs but actively shape development by identifying privacy risks early enough to influence architectural decisions. Organizations that view DPIAs as strategic tools rather than compliance exercises often discover that this structured analysis improves not only privacy protection but also overall system quality and user trust. The DPIA process also creates natural integration points for emerging "AI ethics" considerations that may extend beyond strict regulatory requirements, allowing organizations to evaluate broader societal implications alongside compliance concerns. Regular reviews and updates of DPIAs throughout the AI lifecycle ensure that privacy considerations remain central as systems evolve and new risks emerge.
Implementing Privacy-Enhancing Technologies
Privacy-enhancing technologies (PETs) have become essential tools for enabling GDPR-compliant AI development and deployment. These technologies allow organizations to derive value from personal data while reducing privacy risks through technical controls rather than solely relying on policy measures. Federated learning represents one of the most promising approaches for privacy-conscious AI, allowing models to be trained across multiple devices or servers without centralizing raw personal data. This technique enables organizations to develop accurate models while keeping sensitive information at its source, significantly reducing data transfer and centralized storage risks. Differential privacy has similarly gained traction by adding calibrated noise to data or model outputs, providing mathematical guarantees against re-identification while preserving aggregate insights essential for machine learning. More mature implementations allow organizations to quantify and control the privacy-utility tradeoff, selecting appropriate privacy budgets based on data sensitivity and application requirements. Homomorphic encryption, though still computationally intensive for many applications, enables computations on encrypted data without decryption, creating new possibilities for privacy-preserving analytics in highly sensitive domains like healthcare and finance. Secure multi-party computation allows multiple organizations to jointly analyze their data without revealing underlying information to each other, enabling collaborative AI development between entities that cannot directly share data due to competitive or regulatory constraints.
Organizations increasingly implement multiple PETs in combination to address different aspects of GDPR compliance for their AI systems. For example, a financial services firm might use differential privacy to protect training data, explainable AI techniques to provide transparent decisions, and secure enclaves for processing particularly sensitive information. The selection of appropriate technologies depends on factors including data sensitivity, application context, risk profile, available expertise, and performance requirements. While implementing PETs often requires significant technical expertise and resources, the investment typically yields both compliance benefits and competitive advantages through enhanced data access and customer trust. Organizations should view PETs not as silver bullets but as components of comprehensive privacy strategies that also include appropriate policies, contracts, and governance structures. The rapid evolution of these technologies demonstrates how regulatory requirements can drive technical innovation when organizations view compliance as an opportunity rather than merely a cost. As PETs continue to mature and become more accessible, they will likely play an increasingly central role in reconciling the data needs of advanced AI systems with the privacy protections mandated by GDPR and similar regulations worldwide.
Documentation and Accountability Frameworks
Robust documentation and accountability mechanisms form the foundation of demonstrable GDPR compliance for AI systems. The regulation's accountability principle requires organizations to not only comply with data protection requirements but also to demonstrate this compliance through appropriate documentation and governance structures. For AI systems processing personal data, this typically includes maintaining comprehensive records of processing activities with detailed information about data flows, purposes, security measures, and retention periods. Organizations should document key design decisions that impact privacy, including algorithm selection, feature engineering choices, and the implementation of privacy controls. When automated decision-making occurs, additional documentation covering the logic involved and safeguards implemented becomes essential. Beyond these baseline requirements, leading organizations maintain detailed records of their AI governance frameworks, including roles and responsibilities, risk assessment methodologies, testing procedures, monitoring processes, and incident response plans. This documentation serves multiple purposes: it demonstrates compliance to regulators if questioned, provides essential knowledge for maintaining and enhancing systems over time, enables effective handovers between teams, and supports internal governance and oversight. The most effective documentation strategies balance comprehensiveness with usability, employing standardized templates, clear version control, and accessible repositories that make information available to those who need it while maintaining appropriate security.
The implementation of formal AI governance structures significantly enhances accountability under GDPR. Many organizations have established dedicated AI ethics committees or review boards that evaluate high-risk applications against both regulatory requirements and broader ethical principles. These bodies typically include diverse expertise spanning technology, law, ethics, and relevant domain knowledge, ensuring multidimensional assessment of AI systems. Some organizations have created new roles specifically focused on AI governance, such as AI Ethics Officers who work alongside Data Protection Officers to provide specialized oversight of algorithmic systems. Regular compliance audits and monitoring processes help ensure that AI systems continue to meet GDPR requirements throughout their lifecycle, particularly important given the tendency of machine learning models to evolve as they process new data. Organizations should implement clear processes for handling data subject requests in the context of AI systems, including mechanisms for providing access to personal data, acting on erasure requests, and implementing objections to processing. The most sophisticated governance frameworks include validation and testing protocols that systematically assess AI systems for privacy risks, bias, and compliance issues before deployment and at regular intervals thereafter. When properly implemented, these accountability mechanisms not only satisfy regulatory requirements but also build the organizational capability to develop more responsible AI systems that earn user trust and avoid harmful outcomes.
The Evolving Regulatory Landscape
The EU AI Act and Its Relationship with GDPR
The EU AI Act represents the most significant regulatory development affecting AI systems since GDPR, creating a complementary framework specifically designed to address AI risks. While GDPR focuses primarily on personal data protection, the AI Act takes a broader approach to AI governance, establishing tiered requirements based on an application's risk level regardless of whether personal data is involved. This creates an overlapping but distinct compliance landscape where organizations must satisfy both sets of requirements for many AI applications. The interaction between these frameworks is particularly evident in high-risk AI systems that process personal data, where GDPR principles like data minimization and purpose limitation apply alongside AI Act requirements for risk management, human oversight, and technical documentation. Beyond high-risk applications, the AI Act's transparency requirements for certain AI systems like chatbots and emotion recognition systems complement GDPR's transparency provisions while extending to scenarios where personal data might not be processed. Organizations developing comprehensive compliance strategies increasingly view these requirements holistically rather than as separate compliance workstreams. This integrated approach recognizes that measures implemented for GDPR compliance often support AI Act requirements as well—robust data governance supports both data minimization under GDPR and data quality requirements under the AI Act, while transparency documentation can address obligations under both frameworks simultaneously.
The AI Act's risk-based approach significantly impacts how organizations prioritize compliance efforts across their AI portfolios. Systems classified as "unacceptable risk" face outright prohibition, affecting applications like social scoring and certain forms of biometric identification. "High-risk" systems, including those used in critical infrastructure, education, employment, law enforcement, and access to essential services, must meet stringent requirements for risk management, data governance, technical documentation, human oversight, accuracy, and robustness. AI systems interacting with individuals, such as chatbots or emotion recognition systems, face specific transparency requirements regardless of risk level. For organizations already implementing GDPR, this tiered approach necessitates new assessment methodologies to classify AI applications according to AI Act risk categories alongside existing GDPR risk evaluations. Leading organizations have developed integrated risk assessment frameworks that address both regulations simultaneously, identifying compliance synergies and conflicts. As implementation timelines for the AI Act progress, organizations are incorporating its requirements into their existing privacy and AI governance programs to create unified frameworks addressing the full spectrum of European regulatory expectations. This convergence of data protection and AI-specific regulation represents a broader trend toward comprehensive digital regulation that addresses not only privacy but also safety, fairness, and transparency—a trend likely to continue as other jurisdictions develop their own AI governance frameworks.
Recent Enforcement Actions and Regulatory Guidance
Recent enforcement actions provide valuable insights into how data protection authorities are applying GDPR requirements to AI systems in practice. Several notable cases have established important precedents: a major credit scoring company was fined for insufficient transparency about how AI algorithms influenced credit decisions; a facial recognition company received significant penalties for collecting and processing biometric data without proper legal basis; and an employment screening service faced enforcement action for automated decision-making without adequate safeguards or human oversight. These cases demonstrate that regulators are actively scrutinizing AI applications, with particular focus on high-impact systems affecting financial opportunities, employment, and other significant aspects of individuals' lives. The enforcement patterns suggest several compliance priorities: transparency about AI processing remains a central concern, with authorities expecting clear explanations of how algorithmic systems use personal data; legal basis requirements are strictly interpreted, particularly for sensitive data and automated decisions; and technical compliance measures must be supported by appropriate organizational governance to satisfy accountability requirements. Notably, regulators have shown increasing sophistication in their technical understanding of AI systems, moving beyond surface-level assessments to detailed examination of algorithm design, training data practices, and validation methodologies. This evolution suggests that superficial compliance approaches are unlikely to withstand regulatory scrutiny, encouraging organizations to develop more substantive governance programs that address the full complexity of their AI operations.
Guidance from data protection authorities continues to evolve, providing clearer direction for organizations implementing AI systems. The European Data Protection Board has issued guidelines on several AI-relevant topics, including automated decision-making, data protection by design, and DPIAs, while national authorities have published sector-specific guidance for AI in finance, healthcare, and human resources. This guidance increasingly emphasizes the need for rigorous risk assessment before deploying AI systems, with particular attention to potential discrimination, manipulation, or surveillance risks. Authorities have clarified expectations around algorithmic transparency, suggesting that while technical complexity does not excuse opacity, explanations should be appropriate to the audience—from simplified overviews for general users to detailed technical information for regulators. Guidance on training data has emphasized the importance of representative, accurate datasets and the need to continuously monitor for bias, with several authorities suggesting that organizations should document diversity metrics for training data used in high-impact applications. With respect to automated decision-making, regulators have taken increasingly skeptical views of nominal human involvement, suggesting that meaningful human oversight requires genuine capacity to change decisions rather than merely rubber-stamping algorithmic recommendations. Organizations should monitor this evolving guidance closely, as it often signals enforcement priorities and provides valuable insights into how authorities interpret broadly-worded GDPR provisions in specific AI contexts. Those that align their compliance programs with regulatory guidance typically face reduced enforcement risk and can more confidently innovate within established boundaries.
Case Studies of Successful GDPR-Compliant AI Implementation
Financial Services: Credit Scoring and Fraud Detection
A major European banking group's implementation of GDPR-compliant credit scoring algorithms demonstrates how thoughtful compliance approaches can enhance rather than hinder AI effectiveness. Facing both GDPR requirements and sector-specific regulations, the bank developed a comprehensive governance framework for its lending algorithms that addresses data protection throughout the model lifecycle. The approach begins with careful data selection, using only information with clear legal bases and documented relevance to creditworthiness while excluding protected characteristics that could create discrimination risks. The bank employs explainable AI techniques including SHAP values and partial dependence plots to understand feature importance, enabling them to provide borrowers with personalized explanations of credit decisions through an intuitive digital interface. This transparency not only satisfies regulatory requirements but has improved customer satisfaction by helping applicants understand how to improve their credit profiles. The system maintains human oversight through a tiered review process where algorithms flag borderline or unusual cases for specialist review, with clear escalation paths for complex situations. Continuous monitoring includes both technical performance metrics and fairness assessments across different demographic groups, with quarterly reviews by a cross-functional governance committee. Perhaps most impressively, the bank has developed a sophisticated process for handling erasure requests that can remove individual data from training sets without requiring full model retraining, balancing individual rights with operational efficiency. This comprehensive approach has not only satisfied regulators but has improved model performance by ensuring high-quality, relevant data inputs and building customer trust through transparency.
In the fraud detection domain, a payment processing company has successfully implemented advanced machine learning while maintaining GDPR compliance through innovative technical approaches. The company's fraud prevention system analyzes transaction patterns in real-time to identify potential fraud, a use case where both speed and accuracy are critical. Recognizing the privacy implications of processing vast transaction datasets, the company implemented a differential privacy framework that adds calibrated noise to aggregated data used for model training, providing mathematical guarantees against re-identification while preserving patterns necessary for fraud detection. This approach allows them to leverage transaction data for algorithm improvement while protecting individual privacy and complying with purpose limitation requirements. The system employs federated learning techniques that develop models across multiple regional data centers without centralizing sensitive transaction data, addressing both data minimization goals and cross-border transfer restrictions. For transparency, the company has developed a layered approach: merchants receive general information about how the fraud detection system works, flagged transactions include specific risk factors, and detailed documentation is maintained for regulatory review. When transactions are declined, customers receive clear explanations and straightforward appeal mechanisms, satisfying Article 22 requirements for automated decisions. This thoughtful implementation demonstrates how privacy-enhancing technologies can enable sophisticated AI applications even with highly sensitive financial data. The company's approach has not only satisfied regulatory requirements but has actually improved fraud detection accuracy by ensuring more diverse training data through privacy-preserving federation across regions that might otherwise have been excluded due to transfer restrictions.
Healthcare: Diagnostic Support and Patient Management
A European healthcare network has successfully implemented AI-powered diagnostic support tools while navigating the particularly stringent requirements for processing health data under GDPR. The organization's approach centers on a comprehensive legal framework that addresses both GDPR and sector-specific healthcare regulations. Rather than relying solely on consent, which could be questioned in patient-provider relationships, they established a multi-faceted legal basis combining necessary processing for healthcare provision (Article 9(2)(h)) with appropriate safeguards including professional secrecy obligations and purpose limitations. The diagnostic AI system was designed with privacy principles from inception, implementing data minimization by using only clinically relevant information and incorporating pseudonymization techniques that separate patient identifiers from clinical data used by the algorithms. For transparency, the organization developed clear communication materials explaining how AI assists clinicians, ensuring patients understand that algorithms provide recommendations but qualified healthcare professionals make final diagnostic decisions. This human-in-the-loop approach not only improves clinical outcomes but also addresses concerns about automated decision-making under GDPR Article 22. The system includes robust security measures including access controls, encryption, and audit trails, with regular penetration testing to verify protection. Perhaps most innovative is the organization's approach to algorithm development, using synthetic data generated from aggregate patient statistics for initial training and testing, substantially reducing privacy risks while still creating effective models. When real patient data is necessary for validation or refinement, the organization implements strict data minimization and purpose limitation controls. This comprehensive approach has enabled the healthcare provider to improve diagnostic accuracy and efficiency while maintaining compliance with Europe's strict health data protection requirements.
A medical research institution has demonstrated how GDPR compliance can be achieved even in the challenging context of using AI for patient outcome prediction and personalized treatment recommendations. The institution implemented a sophisticated governance structure including specialized ethics review for AI projects, detailed DPIAs for high-risk applications, and ongoing algorithmic audits for potential bias or unintended consequences. Recognizing the sensitivity of health data, the organization developed a tiered consent framework that gives patients granular control over how their information is used, from direct care applications to specific research projects, with clear information about AI processing in consent materials. For research applications where comprehensive consent is impractical, the institution works closely with their supervisory authority to implement appropriate safeguards under research exemptions, including robust anonymization techniques, strict access controls, and rigorous ethics review. Their patient management algorithms incorporate explainability from the design phase, using techniques like attention mechanisms and feature importance metrics to help clinicians understand why specific recommendations are made. These explanations are presented through an intuitive visual interface that highlights key factors without overwhelming users with technical details. The organization has invested significantly in bias detection and mitigation, regularly testing algorithms across different demographic groups and adjusting models when disparate performance is detected. This commitment to fairness not only addresses potential discrimination concerns under GDPR but also improves clinical outcomes by ensuring algorithms work effectively for diverse patient populations. By approaching GDPR compliance as an opportunity to enhance rather than constrain their AI systems, the institution has successfully developed advanced healthcare applications while maintaining patient trust and regulatory approval.
Statistics & Tables
This section will include comprehensive statistics on GDPR enforcement related to AI, implementation costs, compliance challenges, and strategic approaches utilized by organizations. I'll create an HTML5 page with responsive tables featuring this data.
Conclusion
The intersection of GDPR and artificial intelligence represents a defining regulatory challenge of our digital era, requiring organizations to balance innovation with robust privacy protections and individual rights. As we've explored throughout this article, successful navigation of this complex landscape demands more than superficial compliance—it requires fundamental integration of data protection principles into the fabric of AI development and deployment. The organizations leading in this space have moved beyond viewing GDPR as merely a constraint, instead recognizing that privacy-conscious approaches often yield more sustainable, trustworthy, and ultimately more valuable AI systems. From implementing privacy by design principles at the earliest stages of development to deploying sophisticated privacy-enhancing technologies that enable innovation while minimizing risk, these leaders are establishing best practices that will likely shape AI governance for years to come. The case studies examined demonstrate that compliance need not come at the expense of effectiveness—indeed, thoughtfully designed AI systems that respect privacy often deliver better outcomes through improved data quality, enhanced user trust, and more careful consideration of potential impacts.
As the regulatory landscape continues to evolve with the implementation of the AI Act and ongoing GDPR enforcement, organizations must maintain flexible, risk-based approaches to compliance that can adapt to new requirements and interpretations. Those that invest in robust governance frameworks, technical safeguards, and transparent practices will be best positioned to thrive in this changing environment. The growing convergence between data protection, AI ethics, and sector-specific regulations suggests a future where responsible AI development incorporates multidimensional assessments that go beyond strict legal compliance to consider broader societal impacts. Organizations that embrace this holistic perspective will not only reduce regulatory risk but will likely gain competitive advantages through enhanced stakeholder trust and more sustainable innovation practices. As artificial intelligence becomes increasingly embedded in critical functions across sectors, the ability to develop these systems in ways that respect fundamental rights while delivering value will separate leaders from laggards in the digital economy of 2025 and beyond.
Frequently Asked Questions
How does GDPR define "automated decision-making" in the context of AI?
GDPR Article 22 refers to decisions based solely on automated processing, including profiling, that produce legal or similarly significant effects on individuals. This includes AI systems that make decisions without meaningful human review, such as automated loan approvals or hiring algorithms. Organizations must either avoid such fully automated significant decisions or implement specific safeguards including human oversight, mechanisms to contest decisions, and transparent explanations.
What constitutes a sufficient explanation of AI decision-making under GDPR?
While GDPR doesn't precisely define what constitutes a sufficient explanation, organizations should provide meaningful information about the logic involved, the significance, and envisaged consequences of automated decisions. The level of detail should be appropriate to the audience, with general explanations for most data subjects and more detailed information upon request. For complex AI systems, this typically involves explaining key factors that influenced decisions rather than comprehensive algorithm details.
Are neural networks incompatible with GDPR due to their "black box" nature?
Neural networks are not inherently incompatible with GDPR, but their complexity creates transparency challenges. Organizations using neural networks must implement additional measures such as post-hoc explanation methods, parallel interpretable models, or enhanced documentation to satisfy GDPR transparency requirements. For high-risk applications, organizations should consider whether more interpretable algorithms might be appropriate despite potentially lower performance.
How does the "right to erasure" impact AI training data?
The right to erasure requires organizations to remove an individual's data upon request, which can affect AI models trained on that data. Organizations must design their data architecture to track training data provenance and implement technical solutions that can remove or nullify the influence of specific data points without retraining entire models. Techniques such as influence functions, incremental learning, and careful documentation of training data sources can help manage erasure requests efficiently.
What's the relationship between GDPR and the new EU AI Act?
GDPR and the EU AI Act are complementary frameworks. While GDPR focuses on personal data protection regardless of technology, the AI Act specifically addresses AI systems based on risk levels, regardless of whether they process personal data. AI systems processing personal data must comply with both regulations. Organizations should implement integrated compliance approaches that address the overlapping requirements efficiently, as many measures satisfy obligations under both frameworks.
Can synthetic data resolve GDPR compliance issues in AI training?
Synthetic data can help address certain GDPR challenges by reducing reliance on real personal data, but it's not a complete solution. If the synthetic data is derived from personal data, the original collection must still have a lawful basis, and organizations must ensure the synthetic generation process doesn't allow for re-identification. Well-implemented synthetic data approaches can significantly reduce privacy risks while still enabling effective model development, particularly for initial testing and validation.
When is a Data Protection Impact Assessment (DPIA) required for AI systems?
A DPIA is required when AI processing is likely to result in high risk to individuals' rights and freedoms. This typically includes systematic evaluation of personal aspects through profiling with significant effects, large-scale processing of special categories of data, or systematic monitoring of publicly accessible areas. Most sophisticated AI systems processing personal data will meet this threshold and benefit from the structured risk assessment a DPIA provides.
How can organizations satisfy data minimization when AI models often require large datasets?
Organizations can satisfy data minimization by carefully selecting relevant features rather than collecting all available data, implementing dimension reduction techniques, using privacy-preserving aggregations, employing synthetic data, and implementing regular data review processes to remove unnecessary information. The principle doesn't prohibit using substantial data when necessary for legitimate purposes but requires thoughtful assessment of what data is truly needed for the specific application.
What are the GDPR implications of using pre-trained AI models from third parties?
When using pre-trained models from third parties, organizations remain responsible for ensuring GDPR compliance. This includes conducting due diligence on how the model was trained, assessing whether it processes personal data, documenting the model's capabilities and limitations, and implementing appropriate safeguards for any high-risk applications. Organizations should obtain contractual guarantees from providers regarding data protection compliance and conduct their own validation testing before deployment.
How do international data transfers rules affect global AI development teams?
International data transfers rules restrict moving personal data outside the EEA without appropriate safeguards. Global AI teams must implement measures like Standard Contractual Clauses with supplementary technical measures, data localization strategies, or privacy-enhancing technologies like federated learning that allow model development without transferring raw personal data. Organizations should assess whether their AI development processes involve restricted transfers and implement appropriate compliance mechanisms based on the specific data flows and countries involved.