Privacy by Design: A Guide to Implementation Under GDPR
Learn how to implement Privacy by Design principles to achieve GDPR compliance, protect user data, and build trust with customers through proactive data protection strategies.
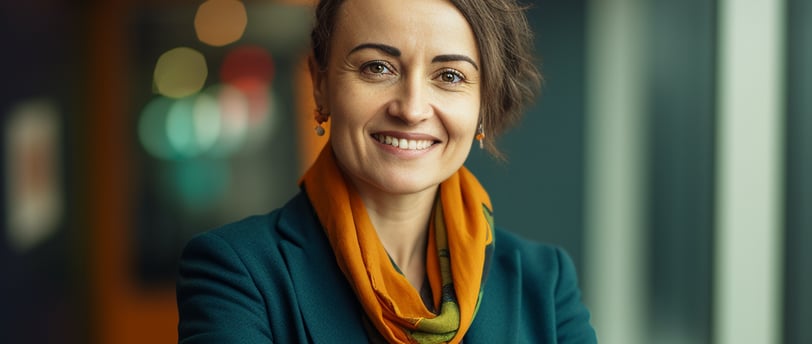
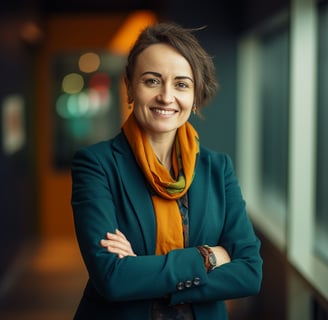
In a world where data breaches make headlines daily and privacy regulations tighten globally, organizations can no longer afford to treat privacy as an afterthought. The European Union's General Data Protection Regulation (GDPR) has transformed privacy from a legal checkbox into a fundamental business requirement, with privacy by design (PbD) standing as one of its cornerstone principles. Rather than retrofitting privacy measures into existing systems—often at significant cost and with limited effectiveness—privacy by design embeds protection into the very DNA of products, services, and processes from inception. This proactive approach not only helps organizations meet compliance requirements but also builds lasting trust with increasingly privacy-conscious users. The implications are far-reaching: reduced breach risks, lower remediation costs, competitive advantage, and sustainable data practices that future-proof organizations against evolving regulations. This comprehensive guide explores how to effectively implement privacy by design principles under GDPR, offering practical strategies, best practices, and real-world examples to help your organization transform privacy from obligation to opportunity.
Enter Privacy by Design (PbD) – a framework that embeds privacy into the design and architecture of systems and business practices. Rather than treating privacy as an afterthought or merely a compliance checkbox, Privacy by Design takes a proactive approach by making privacy an integral part of the system from the very beginning. This concept, originally developed by Dr. Ann Cavoukian in the 1990s, has evolved from a theoretical framework to a legal requirement under the General Data Protection Regulation (GDPR).
This comprehensive guide explores how organizations can effectively implement Privacy by Design principles to achieve GDPR compliance, protect user data, and build trust with customers. Whether you're a data protection officer, privacy professional, or business leader, understanding and implementing these principles will help you navigate the complex landscape of data protection in the digital age.
Understanding Privacy by Design
The Origins and Evolution of Privacy by Design
Privacy by Design was conceived by Dr. Ann Cavoukian, the former Information and Privacy Commissioner of Ontario, Canada. The concept emerged as a response to the growing threats to privacy posed by increasingly sophisticated information and communication technologies. Dr. Cavoukian recognized that privacy could not be assured solely through compliance with regulatory frameworks; instead, it needed to become an organization's default mode of operation.
The framework was initially based on seven foundational principles that aimed to embed privacy into the design specifications of technologies, business practices, and physical infrastructures. Over time, Privacy by Design evolved beyond a theoretical concept to become a widely accepted approach to privacy protection.
The European Union's General Data Protection Regulation (GDPR), which came into effect in May 2018, codified Privacy by Design into law. Article 25 of the GDPR specifically mandates "data protection by design and by default," requiring organizations to implement appropriate technical and organizational measures designed to implement data protection principles effectively.
The Seven Foundational Principles of Privacy by Design
To truly understand Privacy by Design, one must grasp its seven foundational principles. These principles serve as a framework for implementing privacy in a systematic and comprehensive manner:
Proactive not Reactive; Preventative not Remedial: This principle emphasizes anticipating and preventing privacy-invasive events before they happen, rather than offering remedies after violations have occurred. Organizations should take proactive measures to identify potential privacy risks and address them before they materialize.
Privacy as the Default Setting: Privacy should be the default state for any system or business practice. No action should be required on the part of individuals to protect their privacy – it should be built into the system by default. This means that personal data is automatically protected without requiring users to take additional steps.
Privacy Embedded into Design: Privacy should be embedded into the design and architecture of systems and business practices. It should not be bolted on as an add-on after the fact but should be an essential component of the core functionality. This integration ensures that privacy becomes an essential part of the system without diminishing functionality.
Full Functionality – Positive-Sum, not Zero-Sum: Privacy by Design seeks to accommodate all legitimate interests and objectives in a positive-sum "win-win" manner, not through a dated, zero-sum approach where unnecessary trade-offs are made. This principle rejects the notion that privacy must come at the expense of other functionalities or business objectives.
End-to-End Security – Full Lifecycle Protection: Privacy by Design ensures cradle-to-grave, lifecycle management of information. Strong security measures are essential to privacy from start to finish. This involves securing data throughout its entire lifecycle – from collection to deletion or disposal.
Visibility and Transparency – Keep it Open: Privacy by Design aims to assure all stakeholders that business practices or technologies are operating according to stated promises and objectives. Its component parts and operations remain visible and transparent to users and providers alike. This builds trust and accountability.
Respect for User Privacy – Keep it User-Centric: Above all, Privacy by Design requires designers and operators to keep the interests of the individual uppermost by offering strong privacy defaults, appropriate notice, and empowering user-friendly options. This means putting the user at the center of privacy considerations.
Privacy by Design versus Privacy by Default
While often mentioned together, Privacy by Design and Privacy by Default represent distinct yet complementary concepts under the GDPR. Understanding the difference between these two approaches is crucial for effective implementation:
Privacy by Design refers to the integration of privacy measures throughout the entire engineering process of a product or service. It involves considering privacy at every stage of development, from conception to deployment. This approach ensures that privacy protections are built into the system's architecture, rather than added as an afterthought.
Privacy by Default, on the other hand, focuses on ensuring that the default settings of any system or service are configured to provide maximum privacy protection. This means that without any user intervention, the system should collect and process only the personal data necessary for its specific purpose. Users should not have to take additional steps to protect their privacy – it should be the default state.
Together, these two concepts form a comprehensive approach to privacy protection. Privacy by Design ensures that privacy is considered at every stage of development, while Privacy by Default ensures that the resulting systems are configured to protect privacy without requiring user action.
Privacy by Design Under GDPR
Article 25: Data Protection by Design and by Default
Article 25 of the GDPR formally introduces the concepts of "data protection by design" and "data protection by default" as legal requirements. This article mandates that data controllers implement appropriate technical and organizational measures to integrate necessary safeguards into their processing activities.
The regulation requires controllers to implement measures "both at the time of the determination of the means for processing and at the time of the processing itself." This dual-timing requirement emphasizes that privacy considerations must be incorporated from the earliest stages of planning and continue throughout the life of the processing activity.
Article 25 specifically mentions pseudonymization as an example of a technical measure that can be used to implement data protection principles effectively. However, it does not prescribe specific technologies or approaches, recognizing that appropriate measures will vary depending on the nature, scope, context, and purposes of processing, as well as the risks to individuals' rights and freedoms.
Key GDPR Principles Supported by Privacy by Design
Privacy by Design supports and reinforces several key principles of the GDPR:
Lawfulness, Fairness, and Transparency: Privacy by Design helps ensure that personal data is processed lawfully, fairly, and in a transparent manner. By embedding privacy considerations into systems from the outset, organizations can more easily provide clear information about how data will be used.
Purpose Limitation: By designing systems with specific purposes in mind, organizations can ensure that data is collected only for specified, explicit, and legitimate purposes. Privacy by Design helps prevent "function creep" – the gradual widening of the use of data beyond its original purpose.
Data Minimization: Privacy by Design supports the principle that personal data should be adequate, relevant, and limited to what is necessary. By considering privacy from the beginning, organizations can design systems that collect only the minimum amount of data needed.
Accuracy: Systems designed with privacy in mind can incorporate features to ensure that data is accurate and, where necessary, kept up to date. This might include validation processes, regular reviews, and mechanisms for correction.
Storage Limitation: Privacy by Design helps organizations implement appropriate retention periods and deletion mechanisms, ensuring that data is not kept for longer than necessary.
Integrity and Confidentiality: By embedding security measures into systems from the outset, Privacy by Design supports the principle that personal data should be processed securely, including protection against unauthorized or unlawful processing and accidental loss or damage.
Accountability: Privacy by Design helps organizations demonstrate compliance with GDPR principles, supporting the accountability requirement. By documenting privacy considerations throughout the design process, organizations can provide evidence of their compliance efforts.
The Role of Data Protection Impact Assessments (DPIAs)
Data Protection Impact Assessments (DPIAs) are a key tool for implementing Privacy by Design under the GDPR. A DPIA is a process designed to identify and minimize the data protection risks of a project or system. The GDPR requires DPIAs for processing that is likely to result in a high risk to individuals' rights and freedoms, particularly when using new technologies.
DPIAs are an excellent way to systematically analyze, identify, and minimize the data protection risks of a project. They help organizations:
Identify privacy risks to individuals
Identify privacy risks to the organization
Identify privacy solutions to minimize those risks
Integrate privacy solutions into the project
By conducting DPIAs early in the development process, organizations can identify potential privacy issues and design in solutions before significant resources have been committed. This aligns perfectly with the proactive approach of Privacy by Design.
Implementing Privacy by Design in Your Organization
Step 1: Establishing a Privacy Framework
The first step in implementing Privacy by Design is to establish a comprehensive privacy framework within your organization. This framework should provide a structured approach to managing privacy risks and ensuring compliance with regulatory requirements.
Key elements of a privacy framework include:
Privacy Governance: Establish clear roles and responsibilities for privacy management within your organization. This might include appointing a Data Protection Officer (DPO) if required by the GDPR, forming a privacy committee, or designating privacy champions across different departments.
Privacy Policies and Procedures: Develop comprehensive privacy policies and procedures that outline how your organization collects, uses, shares, and protects personal data. These documents should be clear, accessible, and regularly reviewed and updated.
Privacy Risk Management: Implement a systematic approach to identifying, assessing, and mitigating privacy risks. This should be integrated with your organization's overall risk management framework.
Privacy Awareness and Training: Ensure that all employees understand the importance of privacy and their role in protecting personal data. Provide regular training and awareness programs tailored to different roles within the organization.
Vendor Management: Establish processes for assessing and managing the privacy practices of third-party vendors who process personal data on your behalf. This should include due diligence before engagement and ongoing monitoring.
Step 2: Integrating Privacy into the Development Lifecycle
To truly embed Privacy by Design into your organization, privacy considerations must be integrated into every stage of the development lifecycle. This applies not only to software development but to any project or process that involves personal data.
Here's how privacy can be integrated at each stage:
Initiation and Planning: Conduct preliminary privacy assessments to identify potential privacy implications. Define privacy requirements and objectives along with other project requirements.
Design: Translate privacy requirements into design specifications. Consider privacy-enhancing technologies and architectures. Conduct privacy reviews of design documents and specifications.
Implementation: Implement privacy controls as specified in the design. Conduct code reviews with a focus on privacy. Test privacy features and controls.
Testing and Validation: Perform privacy testing, including penetration testing and vulnerability assessments. Validate that privacy requirements have been met. Conduct a DPIA if required.
Deployment: Ensure that privacy settings are correctly configured in the production environment. Provide privacy notices and obtain consent where necessary. Implement procedures for responding to data subject requests.
Operations and Maintenance: Monitor and maintain privacy controls. Conduct regular privacy audits and assessments. Update privacy controls as needed in response to changes in the system or regulatory requirements.
Decommissioning: Securely delete or archive personal data according to retention policies. Document the disposition of personal data.
Step 3: Implementing Technical and Organizational Measures
Privacy by Design requires the implementation of both technical and organizational measures to protect personal data effectively. The specific measures will depend on the nature, scope, context, and purposes of processing, as well as the risks to individuals' rights and freedoms.
Technical Measures might include:
Data Minimization Techniques: Collect only the minimum amount of personal data necessary for the specific purpose. This might involve techniques such as anonymization or pseudonymization.
Access Controls: Implement strong authentication and authorization mechanisms to ensure that only authorized individuals can access personal data.
Encryption: Use encryption to protect personal data both in transit and at rest. This is particularly important for sensitive data.
Data Segregation: Separate personal data from other types of data and implement appropriate boundaries between different categories of personal data.
Audit Trails: Maintain logs of access to and modifications of personal data to detect and investigate unauthorized access or changes.
Privacy-Enhancing Technologies (PETs): Consider technologies specifically designed to enhance privacy, such as differential privacy, secure multi-party computation, or homomorphic encryption.
Organizational Measures might include:
Privacy Policies and Procedures: Develop and implement comprehensive privacy policies and procedures that govern how personal data is handled within the organization.
Staff Training: Provide regular privacy training to employees to ensure they understand their responsibilities regarding personal data.
Vendor Management: Establish processes for assessing and managing the privacy practices of third-party vendors who process personal data on your behalf.
Incident Response Plans: Develop and test plans for responding to data breaches or other privacy incidents. This should include procedures for notifying affected individuals and regulatory authorities as required by the GDPR.
Regular Audits and Assessments: Conduct regular audits and assessments to ensure that privacy controls are functioning as intended and to identify areas for improvement.
Step 4: Documentation and Demonstrating Compliance
Under the GDPR's accountability principle, organizations must not only comply with data protection principles but also be able to demonstrate their compliance. Documentation is therefore a crucial aspect of Privacy by Design.
Key documentation for demonstrating compliance with Privacy by Design might include:
Privacy Impact Assessments: Document the outcomes of privacy impact assessments conducted for high-risk processing activities.
Design Documentation: Maintain documentation of how privacy has been considered in the design of systems and processes, including design decisions made to enhance privacy.
Privacy Policies and Notices: Document the privacy policies and notices provided to individuals, demonstrating transparency about data processing activities.
Consent Records: Where processing is based on consent, maintain records of how and when consent was obtained.
Data Processing Records: Maintain comprehensive records of processing activities as required by Article 30 of the GDPR.
Training Records: Document privacy training provided to employees, including attendance records and assessment results.
Incident Response Records: Maintain records of privacy incidents and the organization's response, including any notifications made to individuals or regulatory authorities.
By maintaining comprehensive documentation, organizations can demonstrate to regulatory authorities that they have taken a proactive approach to privacy protection, consistent with the principles of Privacy by Design.
Challenges and Solutions in Implementing Privacy by Design
While Privacy by Design offers numerous benefits, its implementation can present challenges for organizations. Understanding these challenges and having strategies to address them is crucial for successful implementation.
Common Challenges
Lack of Clear Guidance: While the GDPR mandates Privacy by Design, it provides limited specific guidance on implementation. Organizations often struggle to translate the high-level principles into concrete actions.
Resource Constraints: Implementing Privacy by Design requires investment in terms of time, expertise, and technology. Small and medium-sized organizations, in particular, may find these resource requirements challenging.
Legacy Systems: Retrofitting privacy into existing systems can be technically challenging and costly. Organizations with extensive legacy infrastructure may struggle to implement Privacy by Design principles fully.
Balancing Privacy with Other Requirements: Organizations must balance privacy requirements with other legitimate business needs, such as functionality, user experience, and security.
Rapid Technological Change: The pace of technological change can make it difficult to anticipate privacy risks and implement appropriate safeguards, particularly for emerging technologies like artificial intelligence or Internet of Things (IoT) devices.
Practical Solutions
Start with a Privacy Maturity Assessment: Before attempting to implement Privacy by Design, assess your organization's current privacy maturity. This will help identify gaps and prioritize areas for improvement.
Adopt a Phased Approach: Rather than attempting to implement Privacy by Design across the entire organization at once, start with high-risk or new projects and gradually expand the approach.
Leverage Existing Frameworks: Consider leveraging existing privacy frameworks, such as the NIST Privacy Framework or ISO/IEC 27701, which provide more detailed guidance on implementing privacy by design principles.
Invest in Privacy Training: Ensure that all relevant personnel, particularly those involved in system design and development, receive appropriate privacy training. This will help create a culture of privacy within the organization.
Automate Privacy Processes: Where possible, automate privacy processes such as data mapping, consent management, or data subject request handling. This can help reduce the resource burden of privacy management.
Consider Privacy-Enhancing Technologies: Explore privacy-enhancing technologies (PETs) that can help address specific privacy challenges. These might include anonymization techniques, encryption, or privacy-preserving analytics.
Establish Privacy Champions: Designate privacy champions across different departments to promote privacy awareness and provide guidance on Privacy by Design principles.
Case Studies: Successful Implementation of Privacy by Design
Case Study 1: Financial Services Company
A large financial services company implemented Privacy by Design when developing a new mobile banking application. They:
Conducted a DPIA at the beginning of the project to identify potential privacy risks
Implemented strong encryption for all data transmission and storage
Adopted a data minimization approach, collecting only the information necessary for each function
Built in user controls that allowed customers to easily manage their privacy preferences
Designed the application with privacy-friendly default settings
The result was a successful application with strong privacy protections that enhanced customer trust and exceeded regulatory requirements. The company also avoided costly redesigns that would have been necessary if privacy had been considered only at the end of the development process.
Case Study 2: Healthcare Provider
A healthcare provider implemented Privacy by Design when updating their patient records system. They:
Formed a cross-functional team including privacy, security, legal, and clinical professionals
Created a comprehensive data map to understand all data flows
Implemented role-based access controls to ensure that staff could access only the information necessary for their role
Built in audit trails to track all access to patient information
Developed automated processes for enforcing data retention policies
The result was a system that protected patient privacy while still providing efficient access to necessary information for care providers. The organization also achieved full compliance with both GDPR and healthcare-specific privacy regulations.
Conclusion
Privacy by Design represents a fundamental shift in how organizations approach privacy. Rather than treating it as a compliance burden or an afterthought, Privacy by Design elevates privacy to a core business function that is integrated into systems and processes from the outset.
In the context of the GDPR, implementing Privacy by Design is not just a legal requirement – it's a strategic approach that can deliver significant benefits. By embedding privacy into the design of systems and processes, organizations can enhance trust, reduce risks, improve efficiency, and demonstrate compliance.
While implementing Privacy by Design presents challenges, particularly for organizations with resource constraints or legacy systems, the long-term benefits far outweigh the costs. With a structured approach, clear governance, and appropriate tools and technologies, organizations of all sizes can successfully adopt Privacy by Design principles.
As data protection regulations continue to evolve and public awareness of privacy issues grows, Privacy by Design will become increasingly important. Organizations that embrace this approach now will be well-positioned to navigate the complex privacy landscape of the future and build lasting trust with their customers and stakeholders.
Additional Resources
For readers who want to explore Privacy by Design further, here are some valuable resources:
EU GDPR Compliance Assessments - Professional assessments to evaluate your organization's GDPR compliance status.
Key Principles of GDPR: Safeguarding Data Privacy - A comprehensive overview of the fundamental principles underpinning the GDPR.
The Information Commissioner's Office (ICO) Guide to Data Protection by Design and Default - Detailed guidance from the UK's data protection authority.
European Data Protection Board Guidelines - Official guidelines on implementing Article 25 (Data Protection by Design and by Default).
GDPR Implementation Guidance - Practical advice on implementing GDPR requirements, including Privacy by Design.